Dženan Kovačić - Head of Technology Innovation - BIOptimizers
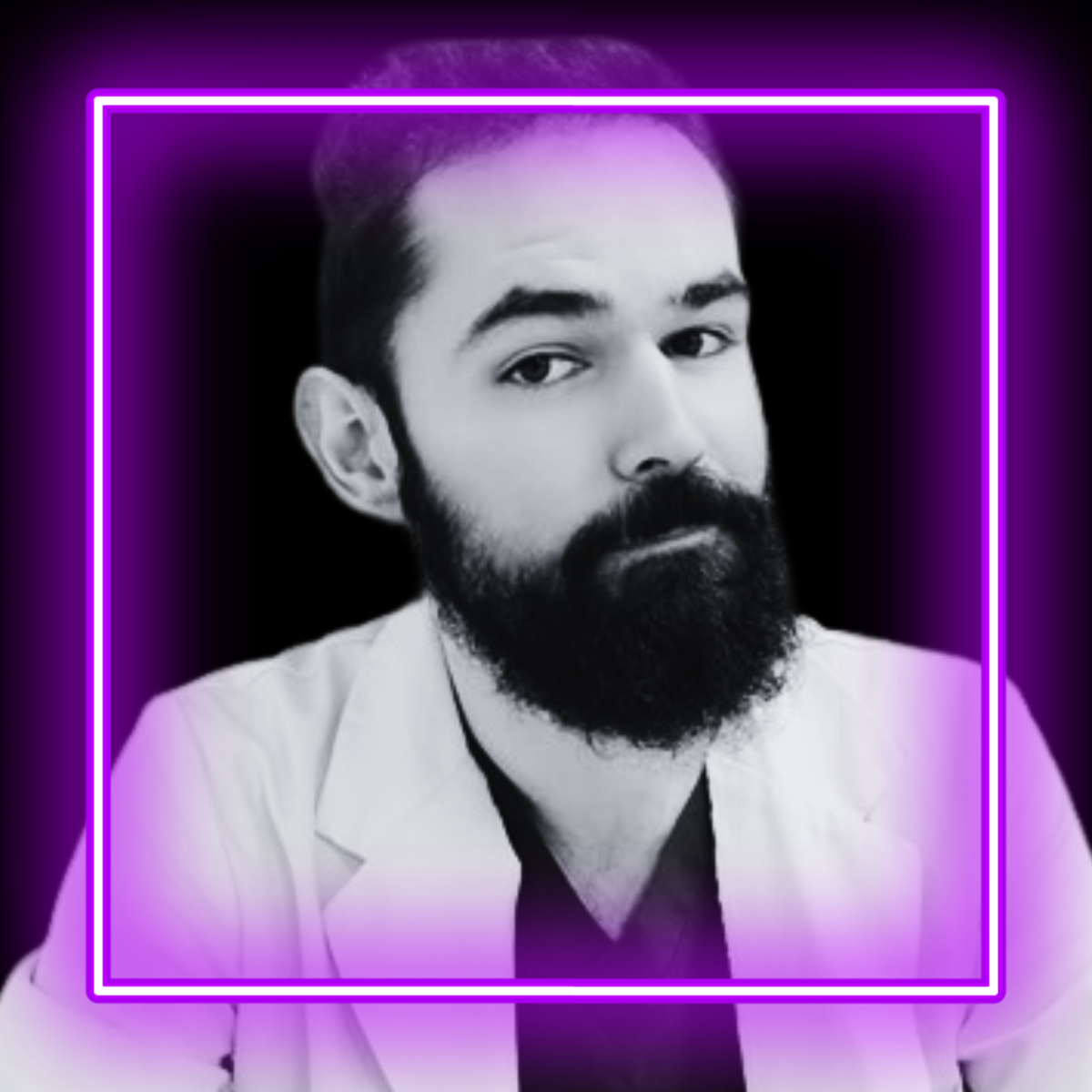
Dženan Kovačić - Head of Technology Innovation - BIOptimizers
Biography
I'm a scientist and entrepreneur with a focus on mRNA and lipid nanoparticle (LNP) research and development. My journey into science began early, and at 19, I was honored to be a finalist at the Falling Walls competition in Berlin for a novel treatment design for human pulmonary tuberculosis. This experience sparked my passion for solving complex problems in medicine. While pursuing a degree in Genetics and Bioengineering at the International Burch University, I found myself drawn to computational biology, systems biology, and bioinformatics due to the limited resources available for traditional lab work. This shift allowed me to explore computational drug design and disease modeling, which became central to my work. When the COVID-19 pandemic began, my colleagues and I contributed research on the role of molecular mimicry in autoimmunity following SARS-CoV-2 infection and vaccination. This was followed by a paper exploring a hypothetical immunological model on the heterogenous effects of BCG vaccination on COVID-19 immunity. During the monkeypox outbreak, we published an mRNA-based multi-epitope vaccine design targeting variola and monkeypox virus proteins. I've had the privilege of presenting our research at conferences like the International mRNA Health Conference and other conference, both domestically and internationally. Today, I lead a team that conducts both academic research and industry projects centered mainly around RNA therapeutic development, LNP development, organs-on-a-chip and synthetic biology.
Interview
NanoSphere: Tell us a bit about yourself—your background, journey, and what led you to where you are today.
Dženan: My journey through the world of medical research or, better said, my affinity for most things “medicine” began rather early – in high school. I went to this medical high school whose curriculum was centered around nursing, however the courses that we were taught peaked my interest almost immediately. Getting to listen to medical doctors teach us about subjects such as pathology, internal medicine, infectious diseases and microbiology, practically hypnotized me. We also had the privilege to do internships at hospitals, shadowing physicians and nurses, where I got to witness numerous complex and debilitating diseases manifest themselves in front of my very eyes. Not to sound morbid, but I never actually wanted to leave since it was all too fascinating. The well-organized chaos where practically all fields of science converge to bring forth what we call the enterprise of modern medicine definitely pulled me in and still hasn’t let go of me. In fact, my first encounter with a medical or scientific problem that relentlessly boggled my mind was when I saw my first patients with pulmonary tuberculosis. Walking out of the infectious disease ward that afternoon, I couldn’t help but obsess over that disease. Learning what I did about how we combated it in the past, I was under the impression that this was a disease of the past, something that would very rarely come up, if ever, and certainly not in a major city hospital. The moment I got home, I started searching the internet for information on tuberculosis, which was the first time I ever encountered scientific publications. Naturally, I had no idea what I was doing, nor did I understand much of what I was reading, but the term ‘latent tuberculosis’ or ‘asymptomatic TB’, became an obsession I wanted to explore further. It was fascinating to me that a bacteria familiar to us for all these years could essentially hijack the immune system to its benefit and persist in the body indefinitely. What was more interesting was the fact that current immunization strategies fail to give permanent and effective immunity against the bacterium, and that treatment of this ‘latent TB’ was incredibly difficult due to the way the bacterium protects itself when ‘hiding’ in the lung tissue. High school then became more of a chore than it did anything else. I just wanted to know whether there was anything that could be done to eliminate the bacillus from the body and, by the time I went on to study Genetics and Bioengineering at the International Burch University in Sarajevo, I already knew exactly what I wanted to devote my time there to. Unfortunately, the labs there did not have the biosafety capabilities to perform research on such a pathogen, and as a freshman nobody really took any of this seriously, which was quite frustrating and demotivating at the time. In the absence of practical requirements to perform this research, I stumbled across the field of computational biology, disease modeling and computational drug design and discovery. ‘This is it’, “ I thought. If I could use these tools to somehow validate this idea, which evolved into a potential treatment design for TB, maybe I could get an opportunity to do this research somewhere else. Obviously, I had no idea just how difficult this field is to get into, especially since I had minimal experience in programming and overall understanding of high-level biology, let alone how to represent it on a computer. To compound these difficulties further, we didn’t really have computational biologists in our academic community, so I was rather isolated in my mission, and somewhat made fun of in the beginning. Nobody around me believed that computers could be used to study something as complex as tuberculosis, nor that anything I could possibly do with computational biology – at least at my education stage at the time – was possible. Stubborn as I was, I decided to ignore such comments and give it a shot. By late March of my freshman year, I was ‘confident’ that I had something in the realm of plausibility. A treatment design that combines eukaryotic vaults – a rather obscure cellular organelle discovered by Leonard Rome and colleagues in the 80s, and small molecule inhibitors of genes responsible for mycobacterial latency mechanisms. With great confidence at the time, I thought I had handled both the drug delivery problem and the drugs themselves, so I decided to apply for the Falling Walls competition that was held locally in Sarajevo. Miraculously enough, the jury liked my presentation and decided to give me 1st place, meaning that I would get to attend the International Conference for Future Breakthroughs in Science and Society in Berlin, and be among the 100 Falling Walls finalists that will present their work in front of a jury comprised of some truly remarkable scientists. The entire conference was a remarkable experience, and I got to connect with incredible people that really motivated me to pursue this work further upon coming back to Sarajevo, with a reinvigorated desire to push forward with computational biology and drug discovery. Soon after I returned from Berlin, the COVID-19 pandemic quickly swept the world, meaning that any possibility of focusing resources towards TB research was understandably non-existent. However, to not stay idle and sucked in by the world of at-home online classes, I used the time spent in quarantined to consume as much knowledge as possible in computation biology and expanded further to immunology and immunoinformatics. Once again, school became more of a chore than anything, and the primary focus became devouring knowledge in these fields. During the pandemic I managed to publish two papers using computational biology, one concerned with how SARS-CoV-2 could trigger autoimmune reactions through molecular mimicry, and another highlighting the possible mechanisms by which the tuberculosis BCG vaccine could render the immune system more competent at combating COVID-19 through heterologous immunity, which were the first two papers I ever published. The moment I heard about mRNA vaccines and Kariko’s work during the pandemic, I was immediately drawn to the concept and later to the field. My immediate thought was “Well, this could be designed and tested on a computer! We’re just playing around with sequences here, and that’s workable!”. The desire to develop mRNA vaccines and therapeutics led me down a rabbit hole of artificial neural networks, high-level bioinformatics and coming up with creative solutions on data integration, interpretation and disease modeling. During the Monkeypox scare, we managed to publish our first mRNA vaccine design for the virus, which was a significant step forward, and reassurance that we were on the right path when it came to this field. Down the line, I managed to get funding for my work and now run a small team of very high quality individuals, where we now work on mRNA immunotherapy development for adenocarcinomas in our university lab facilities. To this end, we combined comprehensive computational frameworks to inform our research directions and facilitate drug design, which we validate through exhaustive wet-lab pipelines that include organ on a chip technology. Thus far, it has been a fascinating, humbling and exciting road!
NanoSphere: To what extent can we currently rely on AI for drug delivery optimization? Can you provide some practical examples for our wet lab colleagues to help them understand its wider applications? Additionally, what are the biggest challenges preventing broader adoption of AI in nanomedicine?
Dženan: The topic of AI in nanomedicine is certainly a complex one. I would break down the problem into two main categories. The first one is the nature of the RNA itself, particularly if the constructs code for some multi-epitope synthetic proteins. Naturally, the relationship between lipid nanoparticle (LNP) encapsulation of nucleic acids depends also on the characteristics of the nucleic acids themselves, so both aspects need to be factored in. The second part pertains to studying LNP formation computationally, and its interactions with cellular membranes of various compositions. I’ve seen numerous attempts at creating machine learning-driven algorithms for things like lipid screening and mRNA construct optimization, all of which carry enormous potential to expedite and somewhat simplify the process of creating not just the lipid-based delivery vehicles, but also RNA constructs themselves. The problem with RNA is that it’s biophysically incredibly dynamic! We don’t yet understand the full spectrum of its behavior within the cellular environment, and even implementing tools such as Molecular Dynamics carries significant limitations for the types of RNA constructs that we would therapeutically be interested in. The space of possible 3D conformations for these molecules is vast, and numerous physical forces influence their behavior once they reach the cell, and that’s without even factoring in stochasticity and quantum effects. This is the case for naturally occurring endogenous RNAs, so just imagine how complex it is to computationally represent an mRNA construct that simply doesn’t exist naturally, which would be the case with multi-epitope mRNA vaccines. On its own, assessing LNP formation and its interactions with cellular lipid bilayers has come a long way, but more work lies ahead. I’ve found tools such as AMBER to be particularly useful in studying LNP dynamics. Yes, it’s computationally very intensive and often time-consuming, but it allows for a lot of good preliminary work on the stability of your lipid compositions. This approach holds a major advantage over running constant encapsulations in the lab on dozens or hundreds of formulations, along with all the characterization steps that go into assessing LNP properties, especially in a resource-limited environment. For example, we are currently working on two separate LNP designs for cell-specific delivery. The actual design itself is informed by extensive computational pipelines, which include screenings for candidate lipids, followed by LNP formation simulations and their interactions with the membrane compositions of the target cells. Considering that we are trying to make these LNPs cell-specific, adding small non-lipid motifs to the formulations is also something that can be represented through computational means, thereby allowing us to paint a clearer picture of what to expect experimentally. These algorithms, whether you are using open-source software or have developed in-house algorithms/commercial software, are certainly not perfect. However, they can help answer a fundamental question: “Does this approach make any possible sense?”. It’s better to try and fail on a silicon chip than in the wet lab, especially if you are dealing with a novel, untested drug delivery vehicle. A major challenge with adopting AI in the world of RNA therapeutics is certainly the misunderstanding of what AI does. At a certain point, implementation of AI in scientific research becomes more of a black box than science itself, which is something researchers need to be aware of. Perhaps the most important aspect of AI implementation in RNA therapeutics is not the AI itself, but discerning hype from reality, and truly understanding what an algorithm is doing and what are its down-to-earth limitations. To trust, but verify, and keep verifying, is definitely a prudent way to go considering the stage we are at with these neural networks. We all certainly want to live in a world where problems can be solved rapidly and with minimal time and financial cost, but that requires us to align this technology to the highest possible degree with our goals in research. To do that, and this is something my team and I are trying to do, one can test AI-informed LNP and RNA designs in the lab for the sake of establishing a feedback loop of data that algorithms can then further ‘learn’ from and optimize their outputs towards higher informativeness. Challenges and issues aside, however, AI implementation has completely transformed our work on many levels, both in the context of workflow simplification, cost savings and higher-resolution insight. I’m particularly excited about molecular dynamics algorithms for studying synthetic RNA constrcuts, particularly when it comes to mRNA vaccines. Similarly to how AlphaFold ended up being an incredible success story when it comes to the protein folding problem, the 3D nature of RNA requires similar efforts. To assess aspects such as translation efficiency, RNA stability and its ultimate behavior once it reaches the cytosol, AI could have an incredibly important role to play. Truly the most exciting application of AI in my opinion is data integration, data analysis and data interpretation. Now, AI-guided lipid library screening and construct optimizations, along with construct design itself, represent domains where these algorithms can truly shine, and they already are! However, over-reliance on AI-guided workflows can be a double-edged sword that researchers need to pay attention to. Therapeutic design is a high-stakes game and having these tools can be incredibly helpful when implemented correctly. Sooner or later, we’ll collectively figure out how to make the most use out of them.
NanoSphere: What is your latest breakthrough in computational drug design & discovery (mRNA & LNPs)? Can you share more details about it, and if published, provide a reference?
Dženan: Currently, we are working on a therapeutic design for triple-negative breast cancer (TNBC), comprised of novel LNP compositions for targeted delivery aimed at delivering a multi-neoepitope mRNA construct to elicit a neoantigen-specific immune response. Now, such a therapeutic design is very complex and time-consuming to experimentally test in vitro, and we aren’t very keen on using animal models unless we are sure that it’s worth it. Thus, we opted to use agent-based modeling, building on works previously published in the domain of TNBC simulation, and see whether the approach would be worth wet-lab experimentation. To this end, major challenges needed to be addressed. Firstly, simulating mRNA translation in silico is very difficult in this context, let alone adding it as a relevant factor in a multi-agent simulation that’s coupled with a quantitative systems pharmacology model. Another problem was that current models are limited in scope and not optimized for studying the fate of the multi-epitope protein that the mRNA codes for, particularly in the context of MHC processing. Additionally, simulating receptor expression and cytokine secretion both by immune cells and cancer cells is quite difficult to do in a holistic way, mostly due to computational cost and emerging complexity when running such simulations. After numerous attempts at optimizing existing models and upgrading them to factor in these details to the highest extent that we could, we managed to build an agent-based model that addresses these problems. Indeed, we have breakthroughs on the side of in vitro disease models and LNP formulations, but building this agent-based model was a true challenge, as we wanted to make it widely available for research use, both in the context of TNBC immunotherapy development, and in a broader context. Introducing mRNA therapeutics to an agent-based cancer model that combines quantitative systems pharmacology, at least to my knowledge, has not been done before. We are now working on further optimizing this model and validating whether its results match in vitro and in vivo experiments. This work is currently under review as a chapter of a monograph entitled “On the Role of Computational Tools in RNA Vaccine and Therapeutic Design”, which we expect to be published soon.
NanoSphere: If there’s one key message or insight you’d like to share with readers for the future of AI in nanomedicine, what would it be?
Dženan: Standardization is key. Currently, we are in quite an exploratory and somewhat chaotic chapter when it comes to harnessing AI in nanomedicine development. In fact, we are in a chaotic chapter when it comes to AI overall, and this is quite normal. It’s important to stay rational and humble, particularly in conversation of what this technology can do for us and our efforts to design better, safer and more efficient medicines. Computational biology is generally a mess to navigate, necessitating the establishment of something at least resembling a standardized pipeline. Perhaps not across all domains, but certainly ones that can have as much of an impact in the real world as therapeutic development does. Richard Feynman summarized this beautifully, and I firmly believe that this applies to our current dance with AI and nanomedicine overall: “For a successful technology, reality must take precedence over public relations, for Nature cannot be fooled”.
Dženan`s references: